On Friday, April 26th at 2 PM EST, PICS will host a colloquium with Neel Dey, Postdoctoral Associate in the Massachusetts Institute of Technology Computer Science and Artificial Intelligence Laboratory. This colloquium will be held in-person in PICS 534 with refreshments provided.
Title
Representations Learnt from Synthetic Volumes Enable Training-free Medical Image Analysis
Speaker
Neel Dey, Postdoctoral Associate in the Massachusetts Institute of Technology Computer Science and Artificial Intelligence Laboratory
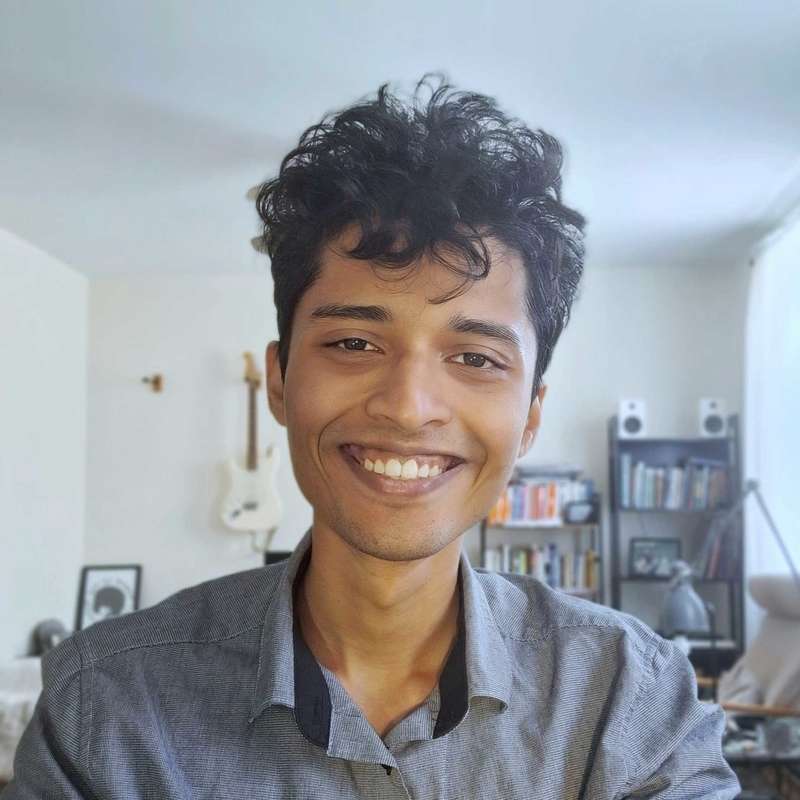
Abstract
Current medical image analysis projects involve months to years of data annotation and custom technical development. This talk introduces methods to train networks that generalize out-of-the-box to new modalities, anatomies, and datasets all without retraining for the specific use case. Our key contributions include (A) generative models driven by biomedical shape priors that synthesize wildly variable training data, and (B) a multi-scale dense representation learning algorithm that leverages the synthetic data to learn contrast-invariant representations. We will show that a single U-Net pretrained in this manner can then extract features that enable state-of-the-art 3D multimodality image registration and can also serve as a general-purpose foundation model for few-shot segmentation across arbitrary biomedical datasets. We will also briefly demonstrate translational applications of the proposed methods to ongoing studies of disordered pregnancies in fetal and maternal MRI.
Bio
Neel Dey is a postdoctoral associate at MIT CSAIL with Polina Golland and received his PhD from NYU advised by Guido Gerig. His research is motivated by the near-universal data and annotation restrictions in radiology and bio-microscopy studies that result in trained models failing in practice on new datasets, imaging setups, subject distributions, and more. To this end, his research develops learning methods that generalize to new biomedical settings by leveraging generative models, geometry priors, and data-efficient learning algorithms. A full profile is available at www.neeldey.com.